News & Insights
Digital infrastructure: the role of technology in predictive maintenance
This article highlights the impact of digital infrastructure like sensors and analytics on predictive maintenance and the benefits it has for facility operations.
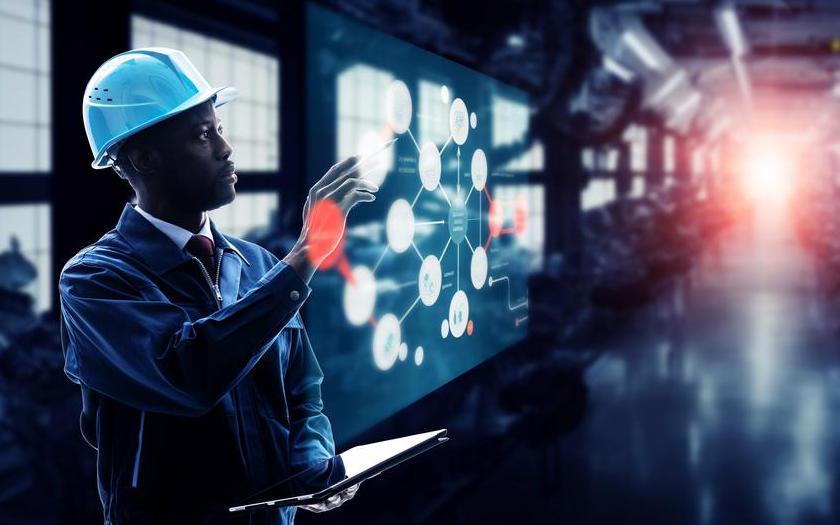
For facilities where efficiency and reliability are paramount, digital infrastructure emerges as a key player, revolutionizing predictive maintenance to optimize the lifespan and performance of critical systems. By leveraging data and digital technology, facility managers can ensure that their infrastructure is not only reliable but also intelligently responsive to ever-changing demands.
This article explores the evolution of maintenance practices, technology solutions for predictive maintenance, and presents a case study demonstrating data-driven technologies in action.
From reactive to predictive: the evolution of maintenance
Facility maintenance is a constant balancing act because production doesn’t stop. There is a whirlwind of daily operations, making it nearly impossible to halt routine responsibilities to concentrate solely on what might happen—which makes it easy to be reactive.
Reactive maintenance models fix systems post-breakdown, much like repairing a car only after it fails to start. This method, while straightforward, often leads to unexpected downtime and higher costs in the long run.
In contrast, a proactive maintenance approach involves regular check-ups and scheduled services to prevent failures before they occur. The goal of proactive maintenance is not merely to maintain but to enhance the system’s reliability through timely interventions. In the car example, this would be like regular oil changes and tire rotations.
Predictive maintenance takes this concept further by integrating advanced technologies such as sensors and data analytics. This approach relies on real-time data to pinpoint potential issues before they manifest as actual problems. For example, if sensors in a car detect that a bearing is wearing out, maintenance can be conducted specifically on that part rather than on components that are not yet problematic. The same applies to facilities. Sensors and dashboards can track parameters like temperature, humidity, water flow, occupancy, and energy usage. Dashboards give facility managers access to a facility’s current performance revealing inefficiencies and predicting systems failures.
The transformation from traditional, reactive maintenance models to proactive and predictive paradigms for facilities is not just a shift in operations; it’s also a strategic, forward-thinking approach to resilience.
Key technologies enabling predictive maintenance
Facility managers have new options when it comes to moving into predictive maintenance. Digital infrastructure can automate predictive maintenance so that it is simply part of the system rather than another disparate element to focus on. Here is a look at the core technologies facilitating this shift:
Networking and interconnectivity
The foundation of predictive maintenance lies in the ability of devices within a facility to communicate seamlessly with one another. This networking is often facilitated by the Internet of Things (IoT) in which sensors and devices are interconnected over a network without requiring human-to-human or human-to-computer interaction.
Interconnectivity allows for the continuous flow of data between devices, which is essential for real-time monitoring. This data exchange enables devices to share status updates, operational metrics, and alerts about potential issues instantaneously. Technologies such as Zigbee, Wi-Fi, and Bluetooth, along with more industrial-focused protocols like Modbus and OPC-UA, establish a robust communication frameworks that supports predictive maintenance strategies. The building management system (BMS) or SCADA system serves as the interface between humans and the data.
Big data and machine learning (ML)
In predictive maintenance, simply collecting data is not sufficient. This data must also be analyzed and turned into actionable insights. Facilities today can collect diverse types of analog signals, such as temperature, pressure, and vibration, through connected devices along with various events such as alarm histories or fault/interlocks.
Once equipment is connected to computer networks, the equipment data can be moved into a higher-level system where it can be processed separately. The goal in analyzing the data is to extract the relationships between sets of process variables. So the more data, the better. This involves big data analytic algorithms, time series data analysis, and machine learning or AI models that identify patterns and anomalies that would be impossible for humans to detect due to the scale of data. For instance, machine learning algorithms can predict the likelihood of a machine’s failure by comparing real-time operational data against historical performance metrics. This capability allows facility managers to proactively undertake maintenance actions based on predictive insights rather than scheduled routines—or worse, unexpected failures.
These types of actions can be computationally expensive to perform, which is why raw data is moved into dedicated environments for analysis.
Cloud computing
Cloud computing provides the scalable and flexible computing resources needed to support the other technologies involved in predictive maintenance. By leveraging cloud platforms, data collected from a myriad of sources can be aggregated into a centralized repository where it can be accessed and analyzed from anywhere in the world. This accessibility is crucial for large-scale operations and enterprises with multiple facilities.
Cloud services, such as those offered by Amazon Web Services (AWS), Microsoft Azure, and Google Cloud, provide powerful tools for data storage, machine learning, and complex analytics. These platforms also offer the reliability and security necessary to handle sensitive operational data. Moreover, cloud computing enables the deployment of advanced analytics models that can learn from new data in real time, continuously improving the accuracy of the predictions and insights they generate.
These technologies—networking and interconnectivity, big data, ML, and cloud computing—are not just enablers; they are the driving forces behind the shift from traditional to predictive maintenance. Together, they form an integrated ecosystem that empowers facility managers to anticipate maintenance needs, optimize operations, and prevent downtime, thereby enhancing the overall efficiency and resiliency of infrastructure.
Enhancements in facility operations through digital infrastructure and predictive maintenance
The integration of digital infrastructure and the resulting predictive maintenance into facility operations has resulted in several significant improvements, enhancing both efficiency and safety. Here are some key enhancements:
- Increased system uptime. Predictive maintenance allows for the identification and repair of components likely to fail, significantly reducing unplanned downtime incidences and ensuring continuous operation.
- Cost efficiency. By allowing for the optimal scheduling of maintenance activities, predictive maintenance helps reduce labor costs and minimizes the use of spare parts. This targeted approach avoids unnecessary maintenance and ensures resources are used more judiciously.
- Enhanced safety. The early detection capabilities of predictive maintenance systems help identify potential failures before they occur and prevent issues from escalating into more serious problems. This proactive approach significantly enhances safety standards by mitigating risks of catastrophic failures.
- Improved asset lifespan. Predictive maintenance techniques help understand the wear and tear on equipment more accurately, allowing for interventions that prolong the operational life of assets. This approach ensures that equipment is maintained in optimal condition, which extends its usability beyond traditional life expectancies.
- Environmental sustainability. Predictive maintenance aligns closely with sustainability goals by ensuring resources are used more efficiently and only as needed. It also helps facilities maintain compliance with environmental regulations by monitoring and reducing waste and resource consumption.
These enhancements not only improve the day-to-day operations of facilities but also contribute to long-term business sustainability and growth. Looking ahead, the continued expansion of networked systems and data accessibility will likely spur further innovations in maintenance technology. Emerging tools could include more advanced diagnostics, real-time performance optimization, and even 3D printing for on-demand replacement parts.
How Salas O’Brien can help
Salas O’Brien helps facility managers create a plan that addresses their specific needs and relieves the pain points within their operations. Not only can Salas O’Brien help you develop a strategic roadmap, but we can also help you justify the investment of digital infrastructure through quantified ROI.
Reach out to our contributors below to talk about your project.
For media inquiries on this article, reach out to Stacy Lake, Director of Corporate Communications.
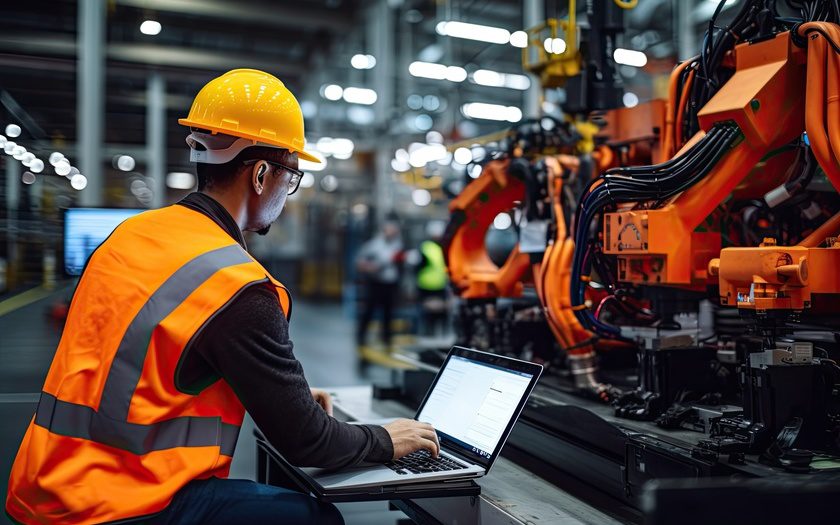
Enhancing equipment maintenance with modern technology
In a pioneering initiative, Salas O’Brien collaborated with an original equipment manufacturer (OEM) to transform traditional maintenance practices using technology. This partnership leveraged digital advancements to develop a “maintenance as a service” model, fundamentally changing how maintenance responsibilities are managed and executed across the industry.
The challenge and solutions
Traditionally, once an OEM sells machinery, the buyer assumes full responsibility for its maintenance, necessitating in-house technical expertise and a stockpile of spare parts. This model places a significant logistical and financial burden on the customer, involving high upfront costs and continuous investment in maintenance personnel and inventory management.
To address these challenges, Salas O’Brien and the OEM co-developed a two-part technological solution:
- Secure communications gateway. Embedded directly into the machinery, this gateway activates upon machine startup and establishes a secure connection to a cloud-based infrastructure.
- Managed Azure cloud solution. This platform enables continuous communication between the field equipment and cloud services and facilitates real-time data transmission and analysis.
Upon delivery and activation at the customer site, each machine begins transmitting data related to its operation and maintenance to the Azure cloud. This data encompasses a wide range of diagnostics and performance metrics that are stored and processed remotely.
Maintenance as a Service (MaaS)
Utilizing the data collected, the OEM now offers a subscription-based maintenance as a service model. This innovative service model alleviates the need for customers to maintain on-site maintenance expertise and inventory, reducing their operational costs. Instead, they pay a manageable monthly fee, and the OEM assumes full responsibility for the maintenance, troubleshooting, and repair of the machinery.
Benefits
For customers. The MaaS model offers a cost-effective solution by shifting maintenance responsibilities to the OEM, who possess deeper expertise and resources. This arrangement not only cuts down on direct maintenance costs but also minimizes downtime and enhances equipment reliability.
For the OEM. This new service model opens additional revenue streams and allows the OEM to offset their cloud infrastructure costs through the maintenance subscriptions. Moreover, real-time connectivity with the machinery enables the OEM to perform predictive maintenance, thereby enhancing service efficiency and profitability.
In action
With over 1,000 machines actively transmitting performance data, the OEM utilizes advanced data analytics, machine learning, and AI technologies to aggregate and analyze equipment performance on a macro scale. This data-driven approach provides invaluable insights into machine behavior and performance trends, informing future design improvements and operational strategies.
Salas O’Brien’s collaboration in developing the MaaS model represents a significant leap forward in how equipment maintenance is conceptualized and delivered. By integrating modern technology into traditional practices, the project not only enhances operational efficiency for the OEM and its customers but also sets a new standard in the machinery maintenance landscape.
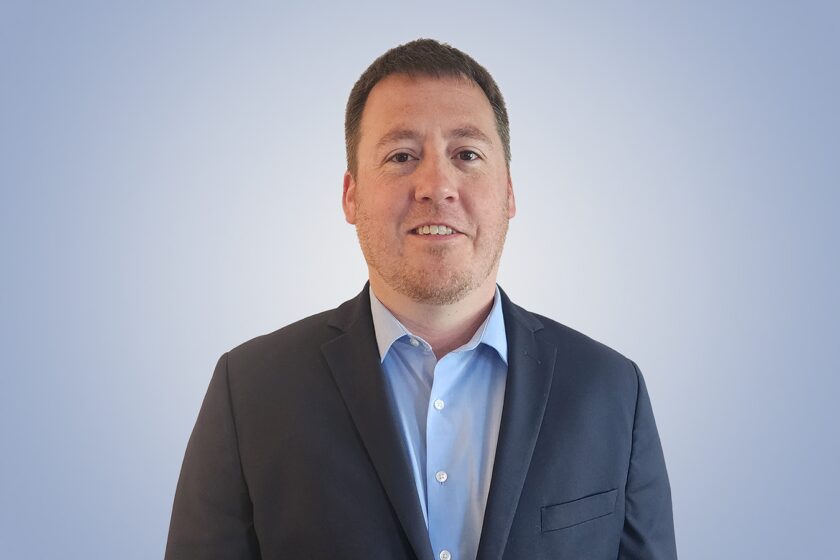
Robert Harman
Robert Harman leads teams that help clients deploy advanced engineering solutions and automations across the chemical, life science, food & beverage, and consumer product industries. With over 15 years of experience, Robert has successfully executed projects from design through programming, commissioning, and startup. He has a Bachelor of Science in Chemical and Biomolecular Engineering from Ohio State University. Robert serves as a Project Engineer at Salas O’Brien. Contact him at [email protected].
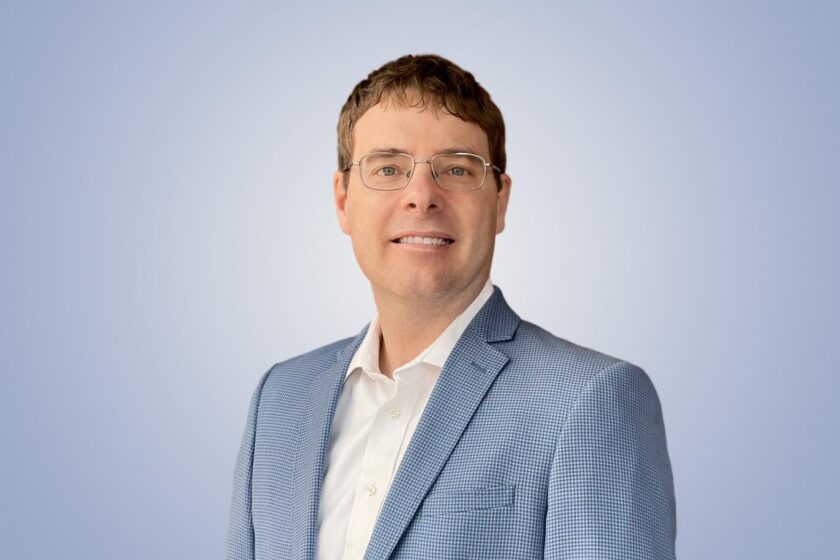
John Glenski, CPM
John Glenski is a leader in digital transformation in the industrial sector with a demonstrated history of providing data-driven outcomes for the world’s largest manufacturers. John works collaboratively with internal and external partners to deliver innovative solutions for smart manufacturing (automation, material handling, and data/information solutions) with a focus on sustainable applications. John serves as a Principal & Senior Director of Automation & Digital at Salas O’Brien. Contact him at [email protected].